Engineering teams can use Applied Intuition’s Synthetic Datasets for on-road autonomy development such as improving rare class detection, constructing validation sets, and even basic image classification. Beyond these on-road applications, teams can also utilize Synthetic Datasets for different machine learning (ML) tasks in a variety of other domains, including off-road and aerial use cases.
During the past year, Applied Intuition has partnered with the University of Southern California (USC), the Army Research Laboratory (ARL), and other academic institutions and research labs to show how its synthetic data can improve ML model performance for specific off-road segmentation and action recognition tasks—two complex tasks where collecting and labeling real data can be difficult, if not impossible. Our team recently attended the SPIE Defense + Commercial Sensing conference, hosted in Washington D.C., to present its work and two publications.
Terrain Segmentation
Unlike most on-road vehicles, off-road vehicles must navigate through difficult terrain with a variety of complex obstacles such as fallen tree branches, rocks, and dense vegetation. Teams commonly use 2D semantic segmentation to enable autonomous systems to navigate these unstructured environments properly.
In 2D images, the semantic segmentation task involves predicting the specific class (such as “vegetation”, “dirt”, or “rocks”) of each pixel in the image. Training ML models that can produce dense information like segmentation is crucial to ensuring that the full off-road domain is captured and vehicles are able to move through it without any problems.

Teams need to train ML models with diverse data that captures the entire off-road domain. Collecting such data can be difficult and more challenging compared to on-road domains. Since unstructured environments are more diverse than on-road settings, data collection runs for off-road environments are often more costly. Additionally, annotating data for segmentation can be expensive and time-consuming, as annotators need to label each and every pixel with proper classes.
In the paper Improved 2D image segmentation for rough terrain navigation using synthetic data, our team used Synthetic Datasets to generate an off-road, fully annotated synthetic dataset that was supplementary to the Robot Unstructured Ground Driving (RUGD) and Rellis3D real-world datasets. Our teams found that mixing this synthetic dataset with the real data provided significant improvements of up to 40% in classes like grass, with almost no degradation in other classes.

Action Recognition
The task of action recognition involves taking in a video stream of data and determining exactly where and when certain activities are occurring. For instance, a team might have an aerial view of a scene and might want to determine what individual actors are doing: are they entering a vehicle, talking to each other, etc.?
Similar to semantic segmentation, the right datasets for training action recognition models are hard to come by. Teams might find it difficult to label training data since they need to know exactly what each actor is doing at each moment in time and even stage certain activities to then record them. Privacy concerns add to the challenge of recording these datasets. It may also be necessary to label items other than activities to create a better scene understanding in the models. For example, knowing the key points of actors or the segmentation of images can be useful auxiliary tasks that help ML models determine what action is taking place. Unfortunately, this only adds to the difficulty and expense of gathering and labeling a robust action recognition dataset.
In the paper Synthetic-to-real adaptation for complex action recognition in surveillance applications, our team generated synthetic data to match the Multiview Extended Video with Activities (MEVA) dataset, which was created as part of the Intelligence Advanced Research Projects Activity (IARPA) deep intermodal video analytics (DIVA) challenge. In particular, our team focused on improving action recognition performance for six difficult activities that involve interactions between different actors:
- People talking on the phone
- People talking to other people
- People entering vehicles
- People exiting vehicles
- People loading vehicles
- People unloading vehicles
Action recognition models must deal with temporal components, making them slightly more challenging than traditional perception models like object detection and segmentation. It is hence important that the synthetic data is consistent with different sensors across time. Our team generated data from various angles and settings to create a synthetic dataset that is fully labeled with 2D and 3D boxes, actor key points, segmentation, and action recognition labels. The study found that mixing the generated synthetic data with the real MEVA dataset provides substantial improvements on these rare classes, showing the utility of using synthetic data to train models even for complex ML tasks.

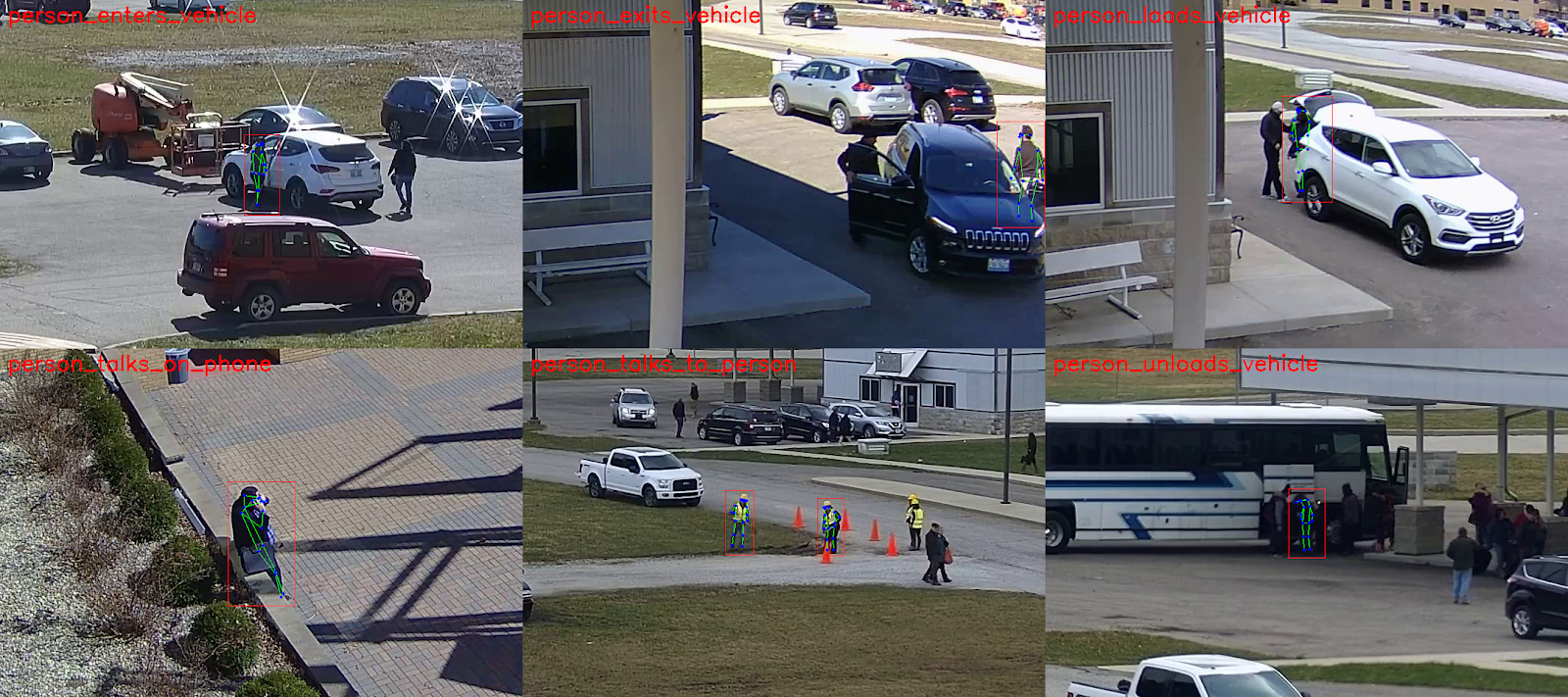
Synthetic datasets are shown to improve models even for challenging, less common tasks such as off-road terrain segmentation and action recognition from aerial assets. However, making this data usable for ML models is not an easy task. Applied Intuition’s Synthetic Datasets help teams generate data that minimizes the domain gap. Teams across industries use Synthetic Datasets in production today, covering a variety of operational design domains and ML tasks.
Learn more about synthetic data for semantic segmentation and action recognition for off-road autonomy development in the papers Improved 2D Image Segmentation for rough terrain navigation using synthetic data and Synthetic-to-real adaptation for complex action recognition in surveillance applications.
Applied Intuition’s Approach
Applied Intuition builds best-in-class digital engineering tools for leading autonomy programs—from the world’s largest automotive original equipment manufacturers (OEMs) to the Department of Defense. Contact us to learn how we can help accelerate your team’s autonomy development efforts.